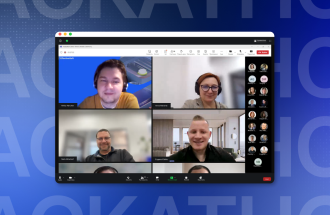
Contact us
Our team would love to hear from you.
This article explores the significance of demand forecasting for businesses—outlining its main types and methods, providing guidance on selecting the most suitable approaches, and offering practical examples from various industries.
Practically every business decision relies on some form of prediction—whether it’s estimating the popularity of a product or service, determining the necessary inventory to satisfy demand, or managing the supply chain to ensure timely product delivery. Accurate demand forecasting minimizes resource waste and prevents issues related to excess or insufficient inventory, facilitates efficient inventory and supply chain management, and supports effective decision-making.
With a wide variety of demand forecasting methods available, companies can effectively tackle complex managerial and strategic problems, allowing them to develop successful marketing campaigns and pricing plans.
Demand forecasting is a challenging process, but it is easier to manage when the following issues are taken into account:
Companies that are just entering the market have limited or no historical data, and therefore can’t base their future predictions on past data. This reduces the available demand forecasting methods.
Company data can become siloed due to inefficient communication and data exchange across different departments. Fragmented data cannot provide a complete picture of the organization’s abilities and position in the market, making it difficult to forecast demand.
Internal processes for predicting demand are generally manageable; however, external factors have a major impact on forecasting and can’t be controlled. Economic fluctuations, market trends, consumer behavior, seasonal demands, social factors, and even legal regulations determine demand and should be factored into predictions.
Demand forecasting types vary based on objectives, data availability, external factors, and internal capacity.
Seasonal products or services forecast and plan short-term activity. By basing their predictions on real-time sales, companies can quickly respond to changes in customer demand and react accordingly.
This type of demand forecasting is useful when planning business growth and expansion. Long-term predictions help set goals and make data-driven decisions on investments, marketing strategy, production, and supply chain, as well as prepare for future demand.
The passive demand forecasting type is based on historical data, with the assumption that past patterns will continue in the future. It offers consistency and is appropriate for companies that prioritize stability over growth. However, this approach tends to be inflexible and fails to account for external factors.
Active forecasting relies on a wide range of data sources and is less dependent on historical data alone. In contrast to passive forecasting, this method is more adaptable and takes into account a variety of external and internal factors, although it is more complex to implement.
The macro-level type of forecasting involves analyzing global and national trends that can affect demand. Factors such as the economy, politics, and even weather conditions can significantly influence the market, customer behavior, and supply chains.
Micro-level forecasting focuses specifically on the industry in which a company operates or the particular customer segment it serves. This approach tailors predictions to a specific type of product and a clearly defined audience.
There are two general groups of demand forecasting methods depending on the approach and the type of data used in the analysis.
These methods rely on quantitative data, including past data on customer demand and sales.
This demand forecasting technique analyzes patterns in historical data to predict future trends. It identifies and explains consistent patterns or systematic variations in datasets, including past sales, market trends, and customer behavior. Access to historical data is crucial for ensuring clear and stable trends and relationships. Trend projections should be adjusted for past anomalies, as these are unlikely to repeat in the future. The trend projection technique is effective for both short- and long-term predictions.
In this statistical type of forecasting, data points are assigned exponential weights, with more recent observations weighted more heavily than older data. The weighted average is then calculated and used to forecast future demand. This demand forecasting technique is most effective for short-term predictions.
The regression demand forecasting model is based on machine learning (ML) algorithms and uses the relationships between past sales data and internal or external factors to predict demand. In this equation, sales data is a dependent variable, while internal and external factors are independent variables that influence future sales and demand. The regression model helps businesses understand their sales performance. It is reliable for short- and medium-term forecasting.
This model determines the relationships between sales performance and external economic factors, such as market trends and economic conditions, and accurately forecast demand for short- and long-term predictions. The demand forecasting technique employs a statistical approach.
This forecasting model relies on three indicators to predict demand: leading, lagging, and coincident. Leading indicators suggest future trends, lagging indicators measure past events, and coincident indicators reflect the current economic conditions. The demand can be predicated by analyzing the movements of these indicators. The barometric model is useful for short-term forecasting.
Diffusion index forecasting is a statistical method used to summarize and analyze common tendencies in economic indicators. By determining upward or downward trends in data, future demand and sales can be predicted.
With this method, comments and company mentions are categorized into positive, negative, or neutral, serving as a basis for social sentiment analysis and future demand predictions. Social network data is a major asset in creating consumer profiles, determining consumer behavior trends, and developing marketing campaigns.
Qualitative methods are less about data and more about human experience and expertise.
This method incorporates opinion polls, surveys, focus groups, and other methods of collecting data from customers. To achieve accurate and relevant results, this should be a systematic, ongoing process. Market research offers a straightforward approach to understanding customer needs and desires, helping businesses customize their marketing campaigns and sales strategies effectively.
Forecasting results rely on the expertise of specialists. This method involves a sequence of surveys, with each based on the results of the previous one. The process is anonymous and aims to provide a unanimous conclusion on future demand. The Delphi method is useful when addressing a specific issue or when historical data is limited or lacking.
Since sales teams interact directly with customers, they have valuable insights into market dynamics, trends, competitors, and effective strategies. This positions them to anticipate how customer demand will evolve.
Many businesses still depend on traditional manual demand forecasting, believing that artificial intelligence (AI) is unreliable and resource-intensive. However, these beliefs are outdated, as advancements in technology and AI have significantly improved the accuracy of forecasting results. Studies have shown that AI-enabled predictions reduce errors by up to 50%. Following are some common AI forecasting techniques.
ML algorithms are powerful tools for uncovering nonlinear relationships in unstructured data. By integrating ML into their forecasting models, companies can enhance accuracy and gain valuable insights to support decisions regarding pricing, inventory, and supply chain management. A significant benefit of ML algorithms is their ability to process vast amounts of data and rapidly adjust predictions based on real-time data.
Neural networks are mathematical models that analyze large, complex datasets to forecast demand. A neural network architecture can consist of different types and various numbers of layers depending on forecasting needs and data characteristics. These models are useful for uncovering subtle relationships among datasets, allowing for even more accurate predictions.
Incorporating NLP enhances the analytics capabilities of demand forecasting models. This technology excels at understanding content and recognizing patterns, making it particularly effective for analyzing unstructured data like customer reviews. By evaluating sentiments expressed in this feedback, NLP can provide valuable insights for predicting future demand.
Image recognition is another valuable technology for anticipating customer preferences. For example, image recognition can be used in the fashion industry, analyzing images on social media to predict future trends.
A sales forecasting tool designed for business planning and analyzing a company’s performance.
Demand prediction is effective in various industries, helping companies optimize operations and support decision-making. Beyond its primary uses in retail and e-commerce, demand forecasting proves valuable in other spheres as well.
This is the primary use case for demand forecasting, as retail and e-commerce companies rely on predictions to inform decisions on everything from procurement to pricing. Companies use demand forecasting techniques to forecast product sales and financial metrics, such as margins and cash balances, as well as monitor and manage inventory and plan for future needs.
Demand forecasting allows companies to anticipate customer needs, plan inventory levels, and mitigate risks related to over- and understocking.
By predicting demand, retail and e-commerce companies can optimize workforce allocation, saving resources and avoiding overspending.
Various forecasting techniques consider different internal and external factors, such as funding and market volatility, that impact a company’s financial health. Accurate predictions can aid in budgeting, financial forecasting, and dynamic pricing strategies.
Future projections facilitate strategic decision-making, including marketing investments and market expansion.
In addition to effective inventory and staff management and strategic planning, demand forecasting has several other practical applications in manufacturing. The analysis of data to make predictions is influenced by the unique characteristics of a specific product category, such as consumer goods, electronics, and automotive. Factors like seasonality, location, and even weather can influence forecasting results and shape company decisions.
By analyzing historical sales data, market fluctuations, seasonal trends, and other economic indicators, manufacturers can optimize their production schedule, reducing waste, saving valuable resources, and improving customer satisfaction.
Accurate demand forecasting in supply chains allows manufacturers to effectively collaborate with suppliers, distributors, and logistics partners, ensuring timely production. At all stages—from planning and procurement to transportation and delivery to partner companies and clients—demand predictions guarantee optimal resource allocation and enhanced operational efficiency.
In fintech, demand forecasting is used to predict future financial and technology trends. By anticipating demand for financial products and services, companies can effectively manage their resources and improve customer satisfaction.
Forecasting techniques are useful in investment portfolio management. Market research and analysis play a crucial role in predicting shifts and anticipating stock performance. By leveraging these insights, investment portfolios can be optimized and adjusted to align with market changes, ensuring maximum value for investors.
By analyzing historical data and customer insights, fintech companies can predict service preferences, meet demand promptly, and tailor their services to individual needs—providing personalized financial plans, loans, and investment options.
Demand forecasting is essential in the healthcare industry, where lives depend on the timely production and distribution of medical products and pharmaceuticals, along with the accessibility of medical care. Key data for valuable predictions include consumer demographics, disease prevalence, seasonal demand, and admission rates.
Forecasting models help pharmaceutical companies estimate the potential demand for certain medicines based on seasonal fluctuations (e.g., allergy season), pandemic and epidemic impacts, and even atmospheric and weather conditions. This enables effective optimization and management of medicine production, supply chain, and inventory, ensuring timely access for healthcare providers and patients.
In healthcare, effective resource allocation is the cornerstone of valuable care and positive outcomes. This includes successful management and distribution of medical staff, inventory, equipment, and capital, ensuring high-quality care and operational and financial efficiency. During peak demand periods, healthcare providers benefit greatly from demand forecasting, which helps allocate resources and attention where it’s needed most.
The hospitality industry relies on demand forecasting to adjust strategies based on the levels of customer interest.
Prices for plane, bus, and train tickets, hotel rooms, and other tourism services can be regulated through dynamic pricing. Based on the number of requests made through search engines or hotel and ticket aggregators, it is possible to predict demand and lower or raise prices for services accordingly.
No forecasting method is perfect. To obtain the most reliable and actionable results, companies should incorporate several methods simultaneously to solve their forecasting problems. The choice depends on various factors, including the industry the business is in, the reason for using demand forecasting, available data, and external factors.
The key determining factor is the maturity and nature of the product or service. For example, forecasting models that are less dependent on historical data are ideal for new products, while mature or declining products typically benefit from leveraging past performance data for analysis and predictions. Industry and product type are also key considerations in selecting the appropriate forecasting model, as this affects whether specific elements, like seasonality or weather, should be incorporated into the model.
Because different problems require different forecasting models, the problem and purpose should be clearly defined, whether it’s short- and long-term sales forecasting for new and current products and services, production and inventory control, financial planning and budgeting, operations and supply chain optimization, risk management, or customer satisfaction improvement.
The selection and accuracy of a forecasting model depend on the availability and reliability of historical data, including sales records, product performance, and customer engagement and behavior. For instance, models that rely on historical data may not be effective for predicting outcomes related to a new product. By incorporating external data sources—such as industry and social trends and economic indicators—companies can significantly improve the accuracy of their forecasts.
Expertise and resources can limit the selection of a suitable model. Advanced models require specific skills that may necessitate hiring reliable IT vendors. An experienced tech partner can also facilitate efficient resource allocation and ensure the timely delivery of forecasting solutions.
Demand forecasting is essential for companies seeking efficient inventory and supply chain management, operations optimization, and improved customer satisfaction. While demand forecasting is complex, effectively addressing challenges—such as choosing appropriate models, continuously refining them, and embracing technology advancements—can lead to greater sustainability, improved decision-making, and increased profits. To learn more about demand forecasting solutions and how they can assist your business, contact our experts.
Demand forecasting is the process of predicting future customer preferences and demand for a specific product or service using forecasting models.
Accurate demand forecasting offers businesses valuable advantages, such as enhanced decision-making, effective risk management, increased operational efficiency, and improved customer satisfaction.
While demand forecasting is a fundamental process for many companies, it does pose some challenges. Common problems include the complexity of forecasting models, lack of in-house expertise, issues with data quality and availability, and the unpredictability of certain events that can impact forecasts. A reliable technology partner can provide the necessary expertise and experience to address these challenges and develop a robust demand forecasting solution.
The demand forecasting process varies depending on the selected models. Generally, it consists of the following steps. First, companies define the problem and set objectives. Then, relevant data is collected and prepared for the analysis. The next step involves selecting forecasting models and adjusting them to fit a company’s goals, which can involve several iterations depending on the results produced. Finally, companies can make further strategic decisions based on the analysis of the results.
Can’t find the answer you are looking for?
Contact us and we will get in touch with you shortly.
Our team would love to hear from you.
Fill out the form, and we’ve got you covered.
What happens next?