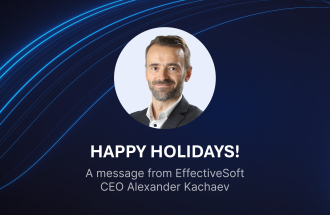
Contact us
Our team would love to hear from you.
Today, effective data governance and data quality plays a critical role as a foundation for any healthcare organization, with the patient as the top priority.
Data analysts hold key positions in extracting value from the vast volumes of data generated through electronic health records, clinical and genomic research, medical imaging and remote patient monitoring tools, and more.
However, healthcare data continues to suffer from errors, inconsistencies, and incompleteness when spread across various isolated systems. Failing to ensure data quality can limit insights and even lead to harmful medical errors.
While data offers substantial opportunities to transform healthcare, ranging from more precise diagnoses to personalized treatment plans, realizing these benefits depends on numerous factors. In this article, we will explain what defines quality healthcare data and offer recommendations for healthcare organizations to ensure the quality of their data.
Data quality has always been a concern for organizations, but the scope of data quality issues has expanded with the growing importance of cloud computing, AI and ML, big data systems and data protection and privacy regulations. Inadequate data is often named as a leading cause of operational errors, inaccurate analytics and poorly planned business strategies. Gartner estimates that poor data quality is costing companies an average $12.9 million annually.
Data quality assessed using various criteria, but the prevalent evaluation factors include:
Maintaining high levels of data quality brings many benefits to organizations across all sectors, including:
Data quality in the healthcare industry plays a pivotal role in delivering safe and effective patient care. In the evolving digital landscape, ensuring the quality of data, information, and knowledge has become paramount.
Poor data quality can have severe consequences, ranging from patient harm and increased healthcare costs to impeding health information exchange, research endeavors, and performance measurement initiatives.
Therefore, the responsibility for maintaining data quality falls on everyone involved in documenting or using health information.
The American Health Information Management Association, the leading authority in health information, has developed a framework for evaluating data quality in healthcare, focusing on four key processes: application, collection, warehousing, and analysis.
Application refers to the utilization of health information management resources and tools.
Collection involves the process of gathering data from various sources, including medical records, electronic health records, and many more.
Warehousing entails securely storing this data in an easily accessible manner.
Finally, analysis encompasses the examination of the data to identify trends, patterns and insights that can inform healthcare decisions.
These processes are critical for effectively managing healthcare information and ensuring accurate and meaningful data analysis.
These processes are evaluated based on ten critical healthcare data characteristics: accuracy, accessibility, comprehensiveness, consistency, currency, definition, granularity, precision, relevancy, and timeliness.
Accuracy is of utmost importance, ensuring that data reflect correct values, are valid, and are associated with the appropriate patient record.
Accessibility means that data is readily and legally available, with robust protections and controls.
Comprehensiveness ensures that all required data items are included, while intentional limitations are appropriately documented.
Consistency ensures the reliability and integrity of data values across different applications. Currency indicates that the data are up to date.
Defining means providing clear descriptions of the data, so that current and future users understand what the data means, and so that each element of the data possesses clear semantics and acceptable values.
Granularity defines the level of detail at which attributes and values of data are specified.
Precision requires data values to be of sufficient magnitude to support the relevant application or process. Relevancy ensures that the collected data hold meaningful significance for the performance of the process or application in which they are employed. Timeliness depends on the specific usage and contextual requirements of the data.
Nevertheless, data quality within individual healthcare organizations, while crucial, is not adequate on its own. Healthcare enterprises may integrate multiple systems that adhere to this data quality model, yet lack the capability to seamlessly share data among all the applications within the enterprise. Therefore, the goal is to achieve enterprise integration which, in turn, requires a metadata approach with established data standards.
Additionally, the healthcare industry faces challenges related to data interoperability between healthcare organizations, highlighting the need for universally accepted data standards. Notable examples of such standards are ones developed by standards development organizations like HL7 and ASTM.
Healthcare quality and safety demand that the right information be available at the right time to support patient care and health management systems. Achieving consensus on essential data content and documentation standards is a necessary prerequisite for ensuring high-quality data in the interconnected healthcare system of the future. Continuous quality management of data standards and content is essential to ensure that the information is both useable and actionable.
Centralized data storage solution for reporting, analysis, and data-driven decision making.
Poor data quality in healthcare can have significant consequences for both patients and healthcare organizations. Let’s examine some of the critical issues that can arise due to inadequate data quality:
Data quality issues can compromise patient care and treatment outcomes. For instance, incorrect or missing allergy information in electronic health records can lead to healthcare providers prescribing medications that trigger severe allergic reactions. This can result in delayed treatment and negative patient experiences.
Inadequate data quality can lead to billing errors, impacting both patients and healthcare providers. Inaccurate or incomplete data can result in coding and invoicing mistakes, leading to claim denials or delayed reimbursement. This, in turn, causes frustration for patients and financial losses for healthcare organizations.
When healthcare professionals encounter data quality issues, they may loose confidence in technology solutions and rely more on manual review processes. This can slow down workflow, reduce productivity, and hinder the adoption of new technologies that could enhance patient care and organizational efficiency.
Accurate data is crucial for developing effective healthcare policies and regulations. Poor decisions and ineffective policies can result if policymakers rely on inaccurate or incomplete data. To understand health trends, identify areas for improvement, and implement evidence-based policies, accurate and reliable data are essential.
Data quality issues can lead to administrative inefficiencies. Healthcare staff may spend significant time manually verifying and correcting data, causing delays and reduced productivity. Ensuring data quality enables healthcare organizations to streamline administrative processes and improve overall efficiency.
To ensure the quality of healthcare data, organizations should consider the following steps:
Implement standardized data collection processes and guidelines, including the use of standardized terminologies, coding systems, and data entry protocols. This ensures consistent and accurate data capture.
Implement data validation checks, quality assurance processes, and regular audits to identify and correct errors, missing data, and inconsistencies. This includes automated validation rules and ongoing data quality monitoring.
Adopt interoperability standards and technologies to facilitate seamless data exchange between healthcare systems and providers. This maintains data consistency and integrity across the healthcare ecosystem.
Provide comprehensive training to healthcare professionals and data custodians on data quality principles, best practices, and the importance of accurate data. Empower them to contribute to data quality in their daily tasks.
Establish clear policies, procedures, and accountability mechanisms for data governance. Define data stewardship roles, quality metrics, and regularly review and update data governance practices.
Perform routine data audits to assess data quality and integrity. Identify and address errors, inconsistencies, and compliance with quality standards promptly.
Implement data quality monitoring tools and processes to proactively identify and address issues. This allows for timely intervention and minimizes the impact of poor data quality on healthcare outcomes.
Participate in data sharing initiatives and collaborate with other healthcare organizations to leverage collective expertise and resources. By sharing data and insights, organizations can enhance data quality through cross-validation and contribute to broader improvements in healthcare.
By following these steps, healthcare organizations can ensure high-quality, informative, and unique healthcare data. This, in turn, supports evidence-based decision-making, improves patient care, and advances healthcare research and innovation.
In the healthcare industry, various data quality tools are employed to ensure the accuracy, completeness, consistency, and reliability of healthcare data. These tools are crucial for identifying and rectifying data errors, enhancing data integrity, and improving the overall quality of healthcare information. Let’s explore some commonly used data quality tools in healthcare:
Data cleansing tools identify and correct errors, inconsistencies, and inaccuracies within healthcare data. They utilize techniques such as data profiling, standardization, de-duplication, and data enrichment to cleanse and enhance data quality. By addressing issues like misspellings, duplicate entries, and formatting errors, data cleansing tools ensure data accuracy and reliability.
Data integration tools facilitate the seamless flow of data across various systems, applications, and databases within healthcare organizations. They consolidate data from different sources, eliminating data silos and enhancing data consistency. This consolidation provides a comprehensive view of patient information and improves data quality.
MDM tools create and manage a centralized master repository of critical healthcare data elements, such as patient demographics and medical codes. They ensure that master data remains accurate and consistent across different systems and applications. MDM tools eliminate duplicate records, maintain data integrity, and serve as a single source of truth for essential data elements.
Data quality monitoring tools continuously assess healthcare data quality in real time. They identify data anomalies, errors, and inconsistencies as they occur, enabling proactive data quality management. These tools establish predefined data quality rules and thresholds, triggering alerts and notifications when issues arise, ensuring timely remediation and data integrity.
Data governance tools assist in establishing and enforcing data quality standards, policies, and procedures within healthcare organizations. They provide a framework for data stewardship, data ownership, and data accountability. Data governance tools support activities like data quality profiling, validation, and lineage tracking, ensuring effective management and sustainability of data quality initiatives over time.
Data quality is paramount in the healthcare sector. High-quality data is indispensable for ensuring safe and effective patient care, enabling informed decision-making, and fostering impactful research. By giving priority to data quality, healthcare organizations can reduce risks, enhance patient safety, optimize healthcare outcomes, and fully harness the potential of data-driven healthcare. It is crucial to acknowledge the significance of data quality and allocate resources towards implementing robust data governance and management practices. In doing so, healthcare organizations can unleash the transformative power of high-quality data to deliver exceptional care and drive ongoing improvements in the healthcare industry.
Turn to EffectiveSoft if you want to ensure the quality of your healthcare data. Contact us to start our partnership.
Healthcare data is derived from various sources, both clinical and non-clinical. Clinical sources include electronic health records (EHRs), laboratory results, imaging systems, pharmacy records, and patient monitoring devices. These systems capture data directly related to patient care, such as diagnoses, treatments, prescriptions, and outcomes. Data from wearables and remote monitoring devices also play an increasing role in chronic disease management and preventive care.
Non-clinical sources encompass insurance claims, patient surveys, administrative records, and social determinants of health. These sources provide contextual information that influences healthcare delivery and outcomes. Additionally, research studies, public health databases, and registries contribute valuable data for epidemiological analysis and healthcare planning.
Maintaining healthcare data quality is challenging due to issues such as incomplete data entry, duplication, and lack of standardization. Inconsistent data formats and terminologies across different systems complicate integration and analysis. Human errors, such as mislabeling or entering incorrect information, further exacerbate these issues, leading to inaccuracies in patient records.
Another major challenge is ensuring timeliness and accuracy amidst high volumes of data generated in real time. Data silos, where information is stored separately across departments, hinder comprehensive analysis and collaboration. Regulatory compliance requirements, such as HIPAA, demand stringent data handling protocols, adding another layer of complexity. Addressing these challenges requires robust governance frameworks and technology solutions. Contact our team to ensure data quality in your organization.
Effective data governance is key to maintaining data quality in healthcare. Establishing clear policies and standards for data entry, storage, and sharing ensures consistency and accuracy. Regular audits and validation checks help identify and rectify errors, while automated tools can flag anomalies and duplicate entries. Adopting standardized coding systems, such as ICD-10 for diagnoses, further ensures consistency across institutions. Leveraging advanced technologies like artificial intelligence and machine learning can enhance data cleansing and integration processes. Additionally, fostering interoperability between systems enables seamless data sharing and minimizes fragmentation. Monitoring KPIs regularly ensures continuous improvement in data quality management.
Can’t find the answer you are looking for?
Contact us and we will get in touch with you shortly.
Our team would love to hear from you.
Fill out the form to receive a consultation and explore how we can assist you and your business.
What happens next?