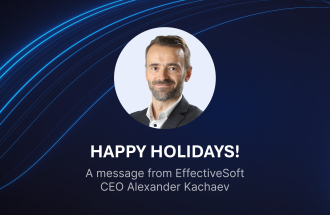
Contact us
Our team would love to hear from you.
This article offers a closer look at machine learning in agriculture explaining how it can be used by farmers and enterprises, benefits, challenges, and machine learning technologies and approaches that help facilitate farming processes.
Machine learning (ML) is a subset of artificial intelligence (AI) that uses algorithms and data to give machines the ability to learn like humans without being programmed. Despite being used interchangeably, ML and AI don’t mean the same. AI is a broader term used for systems that simulate human intelligence processes, while ML focuses on learning only.
There are many applications for ML, including image and speech recognition, natural language processing, predictive modeling, and more. ML solutions are used in healthcare, transportation, finance, e-commerce, agriculture, and other industries, automating processes and improving efficiency. ML allows businesses and industries to make the most of their data, improve decision-making, and provide high-quality services.
These days, agriculture involves making decisions based on huge data sets and in constantly changing environmental, climatic, and economic conditions both locally and globally. Here are some advantages that ML technologies can offer to the industry:
Precision agriculture is an innovative agricultural strategy that can be implemented with the help of machine learning. The ML-based technologies allow enterprises and farmers to improve crop management, maximize crop yields, optimize fertilizer, pesticide, and water inputs, and increase the cost-effectiveness of these processes by employing data from different sources.
Farmers and agricultural businesses generate an immense amount of data, which can be hard to manage without the assistance of ML technologies. Machine learning can efficiently process and analyze lots of complex data that can be used for making informed decisions and building strategies.
Using machine learning solutions, it is possible to predict what factors may have a harmful impact on yield, livestock production, and profits and take measures to reduce their influence or eliminate them completely. In this case, farmers’ expertise is reinforced with the help of ML technologies that consider factors that may be not evident.
This benefit is intertwined with the previous two, as quick data analysis and risk mitigation are some of the aspects that facilitate time management. Moreover, automation of nearly all the processes in agriculture, including crop selection, yield mapping, fertilizers application, weed and insect detection, and more helps save time and money.
Agricultural impact on the environment is an issue that affects the whole world. With the help of machine learning, farmers can optimize water usage, fertilizer and herbicide application, irrigation schedule, livestock grazing, and many other agricultural processes that have a harmful impact on the environment.
Agriculture involves processes and tasks that may be hazardous to humans. Machine learning systems can eliminate the need for farmers to put their lives in danger by automating many tasks, such as working with pesticides and heavy machinery, improving workers’ safety and health and alleviating risks.
Agriculture involves a lot of different procedures and processes that may be hard to follow but become a lot easier with the help of machine learning. Here are some of the use cases for ML technologies in agriculture.
Many different factors affect the price of agricultural products, including location, climate, demand, and government policies. With the advancement of ML technologies, it can become easier to understand price variation, predict costs, and manage risks connected to the factors influencing price. Analyzing this information, farmers can decide which types of crops to plant and whether they need to produce more or cut down on crop production.
Water is an essential resource for agriculture. Since 70% of water use worldwide is used for agricultural production of food, fibers, livestock, and industrial crops, water management is a global concern. Machine learning can help optimize water usage and reduce water consumption by adjusting for soil temperature, moisture and nutrition levels; forecasting weather conditions; and predicting the amount of water that is needed for a crop at a given time. ML combined with other advanced technologies can also be used to detect leaks in irrigation systems.
Crop wellbeing and yield depend, among other things, on soil and its characteristics. Based on soil conditions along with the type of plants, ML systems help decide optimal water and fertilizer input. Thus, with the aid of machine learning technologies, the process of soil monitoring and fertilizing becomes automated, making resource investment efficient, reducing time consumption, and mitigating agriculture’s impact on the environment.
A crop recommendation system using machine learning allows farmers to choose the most profitable and suitable plants based on the parameters and characteristics of soil. This maximizes yield while minimizing resource investment and improving the efficiency of land use.
Timely detection of diseases and immediate action to prevent their spreading is critical for farms that rely on livestock. ML technologies can help monitor animals’ health, daily activity levels, food intake, and other factors that impact production.
Another machine learning application case is tracking livestock feeding habits. Analysis of this information helps disclose animals’ nutrition patterns and abnormalities as well as food influence on their weight and metabolism.
Machine learning can also prevent overgrazing by analyzing aspects such as livestock density, land cover type, and weather to plan when and where animals should graze.
Insects pose a major threat to crops, and early detection is crucial for preventing yield losses. ML systems and algorithms combined with imaging processing technologies can be used to identify harmful insects and analyze data from sensors on temperature, humidity, and other aspects influencing insect activity so that it’s easier to control them.
Another serious threat to farming comes from weeds. ML technologies can detect harmful plants to apply herbicides only to the affected areas instead of all crops. This reduces chemical usage and damaging effects on the environment. Machine learning allows farmers to modify herbicides and make them more effective against persistent weeds.
Machine learning can also automate the weeding process — for instance, an ML-based robot that is designed to target individual unwanted plants and remove broad-leaved weeds using pattern recognition. And that’s just one of the emerging technologies that could be a part of an agricultural revolution.
To maximize yield and increase productivity, specialists must be able to determine what crop to grow on which field and when it is best to harvest it considering weather factors, soil characteristics, fertilizers, and more. Planning the harvest and assigning crops to particular fields can be easier with the help of ML. It becomes possible to map a field’s yield and make crop yield predictions using machine learning by analyzing growth patterns for each crop.
Preservation of desirable characteristics in crops and livestock is not new, but it can take a lot of time and resources. With the assistance of machine learning algorithms and tools, it is possible to quickly and effectively identify and analyze traits that are going to produce the desired results. Though knowledge and experience of agricultural professionals are valuable, machine learning can improve the selective breeding procedure by identifying patterns that may not be obvious to farmers taking into account all the aspects and conditions influencing crops and livestock.
Farmers should not exclude a possibility of a break-in or an accidental destruction of crops by domestic or wild animals, though it may be impossible to detect intruders, especially at distant farm locations. ML-based solutions combined with other AI technologies can identify trespassers and animals and warn farmers, protecting fields and farm facilities.
Despite all the benefits of ML in agriculture, it isn’t widely used just yet. The main obstacles are lack of knowledge (many farmers are used to traditional farming and are not ready to use machine learning or are unaware of the existing technologies), lack of skills to use ML, lack of access to the solutions, and cost. To change the situation, it’s important to raise awareness, educate and train farmers, and continue the development of this sector to make advanced technologies a standard for the industry.
Another barrier is that most machine learning algorithms in agriculture are used on and can be effective only for specific crops in limited regions. With time, the solutions will become more efficient and comprehensive, allowing farmers in different regions to apply them for different crops.
AI subsets and ML technologies, such as deep learning, computer vision, and predictive analytics, can simplify and improve agricultural processes.
A subset of machine learning, deep learning structures algorithms in layers creating an artificial neural network that attempts to simulate the behavior of the human brain. Deep learning algorithms do not require data pre-processing that is involved in ML. They are able to process and analyze unstructured data and make predictions based on this analysis.
The main application cases for deep learning in agriculture are identification and classification of plant diseases, classification of crops, identification of weeds and insects, weather forecasting, and more.
Computer vision is a machine learning field that collects and analyzes data from digital images, videos, and other visual inputs trying to mimic the way human eyes work. The main characteristic of this technology is that it can simultaneously process and gather unlimited amounts of information, unlike humans. Computer vision enables object identification, measuring, movement tracking, and other processes. Computer vision-based technologies can be used to monitor crop health and soil conditions, sort and grade the harvest, monitor livestock health and food supply, count animals, detect abnormal behavior, and more.
Predictive analytics refers to data analysis methods that are focused on forecasting outcomes using historical data together with machine learning, data mining, and statistical modeling to improve decision-making and operational efficiencies and reduce risks.
In agriculture, predictive analytics can help forecast demand, irrigation requirements, crop yield, and weather conditions. Farmers can also rely on this technology to make informed decisions on when and where to plant crops, how to efficiently distribute resources encouraging sustainability, and how to effectively manage risks preventing or reducing potential losses.
ML models can be divided into supervised and unsupervised categories depending on the type of data that is used for their training algorithms.
When we talk about supervised models, there are two types of problems: regression and classification. They are addressed using different training algorithms.
Regression algorithms are used for predictive modeling to determine and analyze the relation between one dependent variable and a series of other variables. For instance, applying regression algorithms, farmers and enterprises can predict yield based on historical dataset, weather and management data, soil information, and other factors. Selective breeding can be approached using regression algorithms to identify crops with desirable traits such as resistance to diseases and other.
Classification algorithms involve identifying the categories of new observations based on independent variables. In smart farming using machine learning, for example, they can be used to classify and filter crops.
Unsupervised machine learning involves algorithms that identify patterns based on similarities and differences in information. Farmers and enterprises can apply these algorithms in crop management to group plants based on different characteristics or according to pre-analyzed conditions under which particular crops are going to thrive.
It’s clear that machine learning cannot replace farmers, nevertheless, it can become a valuable assistant in farmers’ activity. All the existing and potential application cases of machine learning in agriculture provide support for further development of the industry. ML algorithms allow farmers to make well-informed decisions promoting sustainability and automation, as well as reducing the environmental impact of agriculture.
Introducing innovative technologies into processes is a step towards efficiency, smart farming, and precision agriculture. With the help of experts, this step and transition to digitalization can be simple and smooth. Contact our specialists to discuss your project and the ways you can incorporate ML solutions.
Our team would love to hear from you.
Fill out the form to receive a consultation and explore how we can assist you and your business.
What happens next?