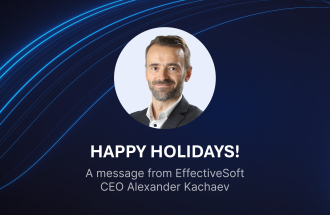
Contact us
Our team would love to hear from you.
With the rise of e-commerce, the existence of many brick-and-mortar stores has been called into question. However, despite cut-throat competition, offline stores have found a way to stay afloat, thanks in large part to the significant contributions of ML to their business operations. Due to its ability to analyze vast amounts of data and enhance work processes, machine learning has influenced numerous industries, from healthcare to transportation to retail. This article specifically focuses on machine learning in retail, shedding light on its advantages, use cases, challenges, and real-life examples of implementing this technology by renowned merchandising businesses.
Machine learning is a subfield of AI that empowers computers to automatically learn from data and previous experience. Arthur Samuel, the pioneer of artificial intelligence (AI), was the first to coin the term “machine learning” (ML) in 1959. According to Samuel, ML is defined as “the field of study that gives computers the capability to learn without being explicitly programmed”. ML involves leveraging algorithms and models. ML algorithms are the instructions that operate on training data to identify patterns and learn from them. Consequently, these algorithms produce ML models which can take the form of a file or computer program. The models capture identified patterns and can be applied to new data. To create an ML model, algorithms are fitted on training data sets, using one of the following approaches:
As the name suggests, this approach requires some human guidance and implies that the algorithm learns by example and observing behavior. To train the algorithm, the data scientist feeds it with labeled or marked datasets that already include the input data paired with the correct output data or target data. By identifying data patterns, the ML algorithm establishes the relationship between inputs and outputs, allowing the classification of new data and making accurate predictions. In case of insufficient accuracy, the model uses human feedback to refine accuracy.
Unsupervised learning involves algorithms that train on unlabeled or raw datasets without output labels. The algorithm runs independently to study data and identify patterns, similarities, and differences to determine how training data is classified or clustered. As an outcome, the machine itself organizes and sorts data without human assistance.
The last approach combines the usage of both labeled and unlabeled datasets to train algorithms. To be precise, an ML algorithm uses small amounts of labeled data to recognize patterns, and then it learns how to label unlabeled data itself. Semi-supervised learning is a good option if you have a lot of unlabeled data, which is typically inexpensive, and crave accuracy in predictions, as well as improved performance.
Machine learning in the retail industry allows retailers to automate and improve key aspects of selling goods and engaging with customers. It plays a crucial role in the industry since it helps retailers analyze vast amounts of customer and transactional data and extract valuable insights that enhance the entire sales cycle. By integrating ML models into their systems, stores can accurately forecast product demand, efficiently manage inventory levels, and optimize pricing strategies in response to market requirements. This results in a refined customer experience, reduced costs, increased sales, and hence revenue growth.
According to Mordor Intelligence, ML is currently the most common AI technology used in the retail industry today, the application of which is estimated to increase from USD 7.30 billion in 2023 to USD 29.45 billion by 2028, with a compound annual growth rate (CAGR) of 32.17% in the forecast period.
Implementing ML technology in retail operations opens up a wealth of opportunities, such as understanding market trends and attracting more customers. Here is a look at what ML can do to benefit your merchandising business:
ML stands as an invaluable tool, powering a company’s customer relationship management (CRM) system. Businesses employ it to analyze customer data, leading to a deeper understanding of their customers. ML aids in predicting what products customers are likely to purchase, which purchasing channels they prefer – be it offline or online through an app or website, and the buying decisions they tend to make. This valuable insight serves as a foundation for developing effective business strategies and relevant marketing campaigns aimed at increasing sales and profits.
With the help of ML, retailers can craft targeted marketing campaigns that center on specific groups of people, considering factors such as age, gender, location, interests, and more. These precisely targeted campaigns optimize advertising efforts and enable companies to customize their products, promotions, or services in accordance with individual buyer preferences. This personalized approach defines the shopping experience and results in increased customer satisfaction and engagement with the brand.
ML algorithms showcase the capability to automate customer service, giving rise to the development of chatbots that serve as proactive customer support and problem-solving tools. Chatbots are designed to provide prompt personalized assistance and are accessible to online shoppers around the clock, 24/7. As a result, waiting times are substantially reduced, and the overall buying experience for customers is greatly enhanced.
By incorporating ML solutions into the sales lifecycle, businesses can automate various routine processes, including product categorization, invoice and inventory management, and customer service, among others. This automation streamlines repetitive and time-consuming tasks, significantly enhancing employee productivity. It enables employees to redirect their efforts toward more creative and complex assignments. As estimated by Statista, ML and AI will enhance labor productivity by 40% in developed countries by the year 2035.
ML empowers retailers to optimize logistics and supply chain management, thereby ensuring timely and uninterrupted deliveries and enhancing the overall customer experience. With ML-based solutions, enterprises can analyze traffic patterns, geopolitical events, and weather conditions to identify time-efficient delivery routes. ML also enables real-time monitoring of supply chain operations, allowing companies to effectively mitigate the impact of supply chain disruptions and provide customers with accurate shipment details for their orders.
Through the integration of ML-powered solutions into their infrastructure, businesses can bolster their security measures by automating risk assessment processes. ML-based solutions facilitate real-time detection and mitigation of potential threats, including financial fraud, phishing, spamming, or malicious bots. Furthermore, businesses can utilize ML solutions to ensure the safety of both customers and employees, prevent theft, and enhance overall security by deploying surveillance cameras and burglar alarms.
The retail industry encompasses various sectors, including fast-moving consumer goods (FMCG), consumer durables, and jewelry, among others. To adeptly analyze and manage the influx of data from these diverse sectors and transform it into valuable business insights for informed decision-making, resulting in increased sales and revenue, the incorporation of ML solutions is essential. Here are the use cases of ML in retail to consider for your trade business:
Retailers widely employ ML algorithms to implement a dynamic pricing strategy, also known as demand or time-based pricing. This strategy involves adjusting the prices of their products or services in response to changes in market demand. Retailers have the flexibility to set and optimize prices by considering metrics like supply, demand, competitor rates, and more. Many businesses turn to dynamic pricing to reduce waste by selling expiring products, resulting in significant cost savings. Additionally, dynamic pricing serves as an effective tool for creating consumer-specific marketing offers, such as discounts, vouchers, and coupons. Overall, a dynamic pricing strategy enables retailers to remain competitive in the market, boost sales of unsold items, and enhance customer loyalty.
To ensure success and achieve business goals, retail companies rely on ML solutions to organize, store, and manage their inventory. This ML-driven approach assists in real-time evaluation of inventory needs, maintaining optimal stock levels to prevent excess inventory and overstocking. It ensures that in-demand items are readily available for consumers while minimizing associated risks and losses. In addition, ML empowers companies to harness predictive analytics using historical data for accurate forecasting of future product demand, a process referred to as demand forecasting. Driven by ML algorithms, demand prediction strongly correlates with weather forecasts, as specific weather conditions significantly influence everyday buyer behavior and product demand.
Another valuable application of machine learning in retail is customer service management, with chatbots playing a significant role. Chatbots simulate human-like conversations with buyers, offering assistance in product recommendations, troubleshooting issues, and answering frequently asked questions. These assistants possess a remarkable feature – if they are unable to provide help, they can redirect the request to a human operator for resolution. Moreover, at the conclusion of the interaction with the purchaser, the chatbot solicits feedback, which aids in measuring customer satisfaction and further enhances the program’s performance. Gartner forecasts that by 2027, at least a quarter of organizations worldwide will deploy chatbots as the primary source of customer service assistance.
Customer segmentation is a crucial step for businesses to better target and tailor their marketing efforts. Powered by ML technology, customer segmentation assists both business-to-business (B2B) and business-to-consumer (B2C) enterprises in categorizing their customers based on specific parameters. B2B companies commonly segment customers by industry, employee count, and location, while B2C companies focus on demographics, encompassing age, gender, location, marital status, shopping patterns, values, and more. By harnessing ML models for customer segmentation, businesses gain the ability to make well-informed decisions regarding the launch of marketing and advertising campaigns, enabling them to target specific audiences, attract more buyers, and retain existing ones effectively.
Retaining existing customers is crucial for every business since acquiring new ones can be five times more cost-intensive, according to Forbes. To reduce the churn rate, businesses employ ML algorithms to analyze historical data and identify patterns that may indicate a shopper’s likelihood to discontinue their purchases from the store. By concentrating on features like purchase history and purchase frequency, companies can pinpoint the most effective strategies for customer retention, consequently reducing the costs associated with acquiring new customers.
ML solutions offer retail enterprises an exciting opportunity to amplify their cross-selling potential. In simple terms, cross-selling entails offering complementary products to customers in addition to their primary purchases. For instance, if a shopper buys a bike, they may be offered a helmet or knee and elbow pads. ML can automatically identify the ideal customers and opportunities for cross-selling, ultimately leading to increased company profits.
Now that you know how machine learning is used in retail, here are some amazing examples of how ML is being implemented by retail giants:
Nike is undoubtedly an unparalleled leader in the realm of athletic footwear and apparel. To maintain a competitive edge in the fiercely contested retail industry, the company has embraced a customer-centric approach, leveraging ML technology to engage with its customers and craft a truly unique experience. For instance, one of Nike’s most recent groundbreaking innovations powered by ML is the introduction of a system that empowers buyers to design their own custom sneakers directly in the store! Furthermore, ML solutions facilitate the collection and analysis of substantial volumes of customer data, enabling the prediction of shopper behavior, anticipation of demand, and the efficient optimization of inventory management. These initiatives ultimately lead to cost reduction, profit maximization, and substantial contributions to Nike’s continued growth.
Another prominent example of the use of machine learning in retail is the fast fashion retailer ZARA, which has turned to ML for various applications. For instance, by analyzing customer preferences and feedback, this clothing retailer generates valuable insights, identifies trends, and designs new products to meet customer expectations. ZARA employs ML algorithms to forecast future demand, allowing for effective inventory management, prevention of stock-outs, and reduction of waste. Furthermore, ZARA has streamlined the process order pickups in physical stores by implementing robots. Shoppers visit the store, enter a pickup code, and a robot delivers the order from the warehouse. In summary, all ML applications in ZARA’s business operations contribute to enhanced productivity, increased sales, and higher revenues.
Equipped with ML solutions, IKEA, the world’s largest furniture retailer, analyzes customer feedback and identifies current design trends to develop new products that align with customer’s preferences. The retailer also integrates ML technology into Virtual Reality (VR) and Augmented Reality (AR) apps, enabling buyers to visualize how the furniture they plan to purchase will appear in their space. This empowers shoppers to make informed decisions about their purchases and contributes to the increased levels of customer satisfaction. As widely recognized, customer satisfaction translates into positive reviews, enhanced credibility, and success in sales.
The final example of the successful implementation of machine learning in retail business processes is illustrated by Walmart. This renowned retailer sells a wide range of goods to customers worldwide, spanning groceries, electronics, clothing, and accessories. It utilizes ML algorithms to optimize all its operations and business processes. Walmart enhances the workflow of its supply chain and logistics through the adoption of the just-in-time method. According to this approach, goods are supplied only when they are needed, reducing waste and boosting the turnover of the inventory. Additionally, Walmart leverages ML to predict product demand, identify substitutes for out-of-stock items, and automate processes, resulting in an improved customer experience and, consequently, increased sales.
ML is a powerful tool that has revolutionized the way retail companies operate today. Used in a wide range of retail applications, from dynamic pricing to customer segmentation, this technology drives business operations and offers numerous benefits for both stores and customers. Leading retailers such as Nike, ZARA, IKEA, and Walmart are implementing ML solutions to process customer data and make informed decisions on how to develop successful marketing campaigns and tailor products to their customers’ expectations and needs. Ultimately, this approach attracts new customers, boosts sales, and significantly enhances the bottom line. If you are considering integrating ML-powered solutions into your business processes and want to start reaping the benefits, do not hesitate to contact us and begin working with the best experts in the field!
Our team would love to hear from you.
Fill out the form to receive a consultation and explore how we can assist you and your business.
What happens next?