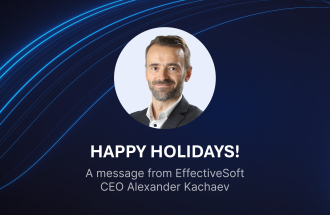
Contact us
Our team would love to hear from you.
To build an effective strategy and drive sales, retailers must consider many variables, such as market tendencies, demand, competition, etc. This task becomes much easier with predictive analytics solutions.
This article explains the basics of predictive analytics, its role and benefits for retailers, and its use in practice.
Data science is a key aspect of decision-making for businesses in various industries. Predictive analytics improves the decision-making process by enabling businesses to look into the future and see what may lie ahead.
As defined by Gartner, predictive analytics is “a form of advanced analytics that examines data or content to answer the question, “What is likely to happen?” Businesses can use predictive analytics to answer this question by identifying patterns in historical and current data. Predictive analytics employs data analysis and artificial intelligence to make forecasts and identify risks and opportunities.
Businesses can create a predictive analytics framework through five general steps:
Each company has requirements for the functionality and desired results of a predictive analytics model, so the first step is to identify the issues that need to be solved and the goals that must be achieved. This consideration determines the choice of the appropriate model.
The next step involves deciding which data will most effectively meet the objectives of the analysis. Companies must also examine all available data flows and select the ones most relevant to the analysis.
Before predictive analysis can be initiated, data must be pre-processed, transforming raw data into clean datasets suitable for further processing, scaling, and feature engineering.
Depending on the problem, objective, and available datasets, different tools and techniques can be used to develop predictive models. Commonly used models include decision trees, regression models, and neural networks.
The effectiveness and accuracy of the model must be assessed through a series of tests. Once the predictive model has been adjusted and its validity confirmed, it can be deployed. Constant monitoring is necessary to ensure the model performs well.
Every retailer wants to be able to anticipate future market trends, outperform competitors, enhance customer experience, and meet customer demands. The decision-making process is heavily influenced by these and other key questions, which can easily be handled with the help of predictive analytics.
Many decisions businesses make involving inventory, merchandising, pricing, marketing, and other store operations rely on data and result in either profit gain or loss. Retail predictive analytics gives companies invaluable insights into the market, consumers, and competitors, which they can use to make long-term plans and improve performance.
Predictive analytics models require access to both historical and real-time (current) data. The former includes information about past events, trends, and patterns, such as purchase history, inventory records, search history, social media interactions, etc. The latter is data that is available immediately after collection, such as seasonal influences, on-shelf availability, promotion campaigns, product data, market conditions, etc.
The use of predictive analytics in retail provides valuable opportunities to not only offer customers a comprehensive experience but also improve profitability. The main advantages of predictive analytics are explained below.
Predictive analytics models provide retailers with relevant information on which to base their strategies and tactics. Based on these forecasts, businesses can develop and adjust marketing, pricing, and other campaigns to boost revenue. Predictive analysis can also be used to determine the long-term popularity of a product or service, allowing retailers to concentrate on those that will deliver the highest profit.
Customers, and their satisfaction with the products and services, are retailers’ main focus. Predictive analytics provides insights that enable retailers to adapt to the needs and expectations of buyers using personalized recommendations and offers, targeting, effective communication channels, and pricing policies, thus improving the customer experience.
With the help of predictive analytics, retailers can optimize and enhance many processes. For example, a retail supply chain depends on many factors, from suppliers and logistics to technology, and any mistake results in delays and lost profit. Predicting what risks may occur, such as when delivery vehicles need maintenance, what can go wrong during transportation, etc., allows retailers to act accordingly and create an efficient workflow.
Every decision a retailer makes should be based on data. Predictive analytics allows retail businesses to support their decisions with insights into potential risks and possible outcomes. The greater the number of variables, the more informed and calculated solutions can be devised. Using predictive analytics insights allows retailers to support their decision-making process with data, gaining a competitive advantage.
By forecasting outcomes, retailers can significantly reduce risks. Predictive analytics models can be used to determine the probabilities of understocking or overstocking, revenue losses, customer churn, and any other events that may influence profits in the future. Being able to anticipate these issues enables companies to develop appropriate risk mitigation plans.
While many industries benefit from predictive analytics, retail examples demonstrate that applying this type of analysis can be a major turning point for retail businesses looking to change their strategies based on data and the insights it delivers.
Demand prediction is one of the most popular use cases of predictive analytics in retail stores. Consumer preferences and needs change constantly and rapidly and retailers must predict these changes to outperform competitors. Using different demand forecasting techniques, businesses can analyze historical and real-time data (sales figures, market research findings, customer reviews, trends, competitor information, consumer requests) to gain insights. Customer demand influences all retail operations, including inventory and supply chain management, stocking and procurement, etc. It is therefore essential to know in advance which products and services to offer to gain customer loyalty and increase sales and profits.
The insights gained using predictive analytics models help retailers create a unique experience for customers. Shopping patterns and customer data, such as purchase history and habits, search history, and preferences, can be used to predict which products will interest them and meet their expectations. And it’s not just about giving the same recommendation to shoppers with similar interests—each individual buyer should receive personal offers customized to their specific needs and desires.
Many factors can help marketers develop campaigns targeted at particular consumer segments, including store navigation intelligence, customer loyalty data, neighborhood demographics, preferences, and purchasing patterns and behavior. To attract new customers in a particular area, retail stores must offer them exactly what they want, when they want it, through the appropriate communication channel.
Predicting which products may appeal to certain customers can aid retailers in developing cross-selling and upselling strategies. Predictive analytics allows retailers to identify consumers likely to buy an item that is more high-end than their usual purchases or add products to complement those already in their carts. In this way, businesses can improve their promotion campaigns while encouraging personalized experiences.
Most retailers typically face two significant problems: understocking and overstocking. With understocking, stores fail to meet customers’ demands, resulting in revenue losses and consumer dissatisfaction, while overstocking leads to product expiration, additional storage expenses, and poor cash flow. Both problems can be solved with the help of predictive analytics, which allows retailers to optimize their inventory management through predictive alert systems that send notifications when they need to stock up on a particular product. Analytics models can use information on seasonal demand, holiday discounts, customer preferences, and other data to predict risks connected to supply chain and inventory management and help retailers take action at the right time.
Setting prices is a complex process that depends on many variables, including product value, market tendencies, competitors’ prices, customer demand, and even time of year or geographical location. In retail pricing, predictive analytics models help retailers build optimal data-driven strategies, determine when to move prices to enhance sales and increase revenue, and respond to market fluctuations promptly.
In retail, the primary focus is always on building and maintaining relationships with customers. However, the market is competitive, and losing buyers is quite common. Churn prediction models are used to identify dissatisfied customers and how likely they are to leave for competitors. Using predictive analytics to change a marketing approach or pricing plan can help retailers retain customers by reminding them about personal discounts and appealing product features, offering different payment plans, and making personalized recommendations.
Planning, launching, and promoting a product or service requires analyzing a significant amount of information from different sources and data flows. Predictive analytics models can be used to estimate the resources and effort required to launch a new product, forecast its success rate, and even estimate the revenue it will generate. This helps retailers mitigate risks, prevent revenue loss, and satisfy customer demand.
Below are some best practices retailers can apply to make the most of predictive analytics:
Know your goals. Predictive analytics begins with defining goals. You can have multiple objectives, but they should be clear and prioritized. For example, opportunities and risks that could have an immediate impact on the business should be addressed first.
Choose relevant data. For accurate predictions, the data for the analysis should be of high quality and directly relevant to the objective.
Use the right visualization tools. All metrics are interrelated, and the applied tool can either help or harm the decision-making process. The results of predictive analysis should be presented in a format that is understandable and clear for all decision-makers.
A robust predictive analytics solution for retail has the potential to completely transform the way businesses develop their strategies. Predictive models facilitate informed decision-making, contribute to customer satisfaction and loyalty, and enable retailers to outperform competitors and optimize inventory, supply chain, and other workflows. Experts in predictive analytics can help your company use data effectively and gain powerful insights to forecast outcomes. If you don’t know where to start, contact us for a consultation and we’ll help you get started!
Our team would love to hear from you.
Fill out the form to receive a consultation and explore how we can assist you and your business.
What happens next?